A guide to preparing and engaging employees for AI assistant adoption. Part III: Relations
20 Sep 2024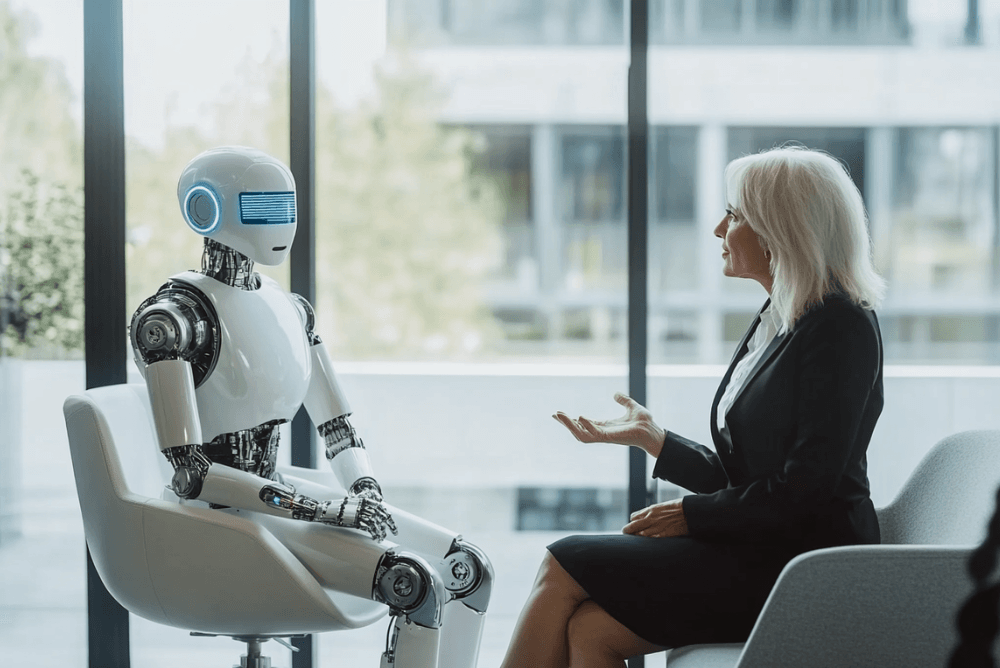
This is the third part of our series of articles, where we share our insights and lessons learned from a recent case study on implementing AI assistants in large companies with 100 to 1,000 employees involved in content creation, as well as in production-focused companies managing thousands of daily service tickets.
We believe our findings can be valuable for both media and production organizations, so if you’re in that loop – jump right in. You will find a short overview of the project and its context here.
This part focuses on relations (which we view as a result of the equation people + system) — highlighting some of the key elements that we believe are crucial for the successful implementation of an AI assistant. The list is not exhaustive – we want to describe what was most crucial (and most challenging to us). These elements are: 1. Transparency and quality control, 2) Supporting people in managing AI, 3) Measuring through feedback, 4) Local feedback.
Also, make sure to check out the other two parts of our case: People and Systems .
1) Transparency and quality control
The word “risk” hasn't come up as frequently in our projects in a long time as it has with the implementation of AI assistants. In this discussion, we’re not only dealing with technological challenges but also legal, financial, and HR-related issues, plus concerns about the quality and usability of the produced content. From the beginning, we understood one thing: to manage these risks effectively, we needed a simple and transparent way to identify the outcomes of AI work.
To address this, we introduced a system that tags all articles and tickets containing AI-generated text, making them easy to locate. But this was just the start. We took it further by maintaining a complete record of every chat interaction, including prompts, responses, sources, and all options and settings used during the process.
Our focus on transparency reduces speculation and rumors, leading to more precise and more informed project management. This ensures that the content we produce is high-quality, useful, and efficient, meeting editorial standards and business goals.
Watch: 6 non-obvious signals showing you might need UX consultancy:
2) Supporting people in managing AI
Do you remember your first conversation with a chatbot? The language and knowledge it displayed were awe-inspiring, as AI can be truly impressive in terms of its capabilities.
However, its shortcomings quickly become apparent when used intensively and professionally. While we may not control many aspects of the AI engine’s behavior, we control our application's user experience (UX).
Examples of specific risks:
-
Quotes: AI tends to paraphrase quotes, which can be problematic. For example, in a news article, you wouldn't want to replace the word "idiot" with "fool" in a quote about someone insulting a public figure. We highlight quotes within the text to ensure they are reviewed manually.
-
Sources: AI doesn’t always follow prompts strictly, sometimes omitting or adding sources. We inform the user of the specific sources the model utilized each time, allowing for proper verification.
So, how can we effectively manage these risks?
-
Identify weaknesses. Before developing your application, test the models through chat interfaces and API interactions. Simulate the tasks that AI will be expected to handle, and pay close attention to any deficiencies that arise.
-
Risk management. Once you’ve identified the weaknesses, you can adapt your application to support users in their AI interactions, reducing the impact of any limitations.
-
Ongoing risk monitoring. Regularly assess these weaknesses in new versions of the AI models. As engines evolve, many problems may resolve themselves.
Examples
Example 1
The model altered quotes despite clear instructions.
We implemented an additional prompt to restore quotes to their original form. Additionally, we flagged quotes within the text so editors could manually verify them. This extra layer of control helped reduce potential errors.
Example 2
The model ignored source-related instructions, raising legal concerns.
We addressed this by attaching a list of sources used by the model, ensuring the sources were accurate and aligned with the original prompt’s requirements.
3) Reviewing AI is boring
Every application must be thoroughly measured, especially one designed as a professional tool. We measure how well the system functions and whether it achieves its intended goals. For example, analytics revealed that AI-assisted articles are written almost five times faster than those written manually.
But measurement is more than just speed—it provides valuable insights into user behavior. This allows us to uncover unconventional scenarios and behaviors that could not have been predicted.
For example, we introduced an “AI-assisted” label for articles where at least one paragraph was generated by AI. After a week, we noticed that the number of such articles was lower than expected. It turned out that editors were bypassing the designated path. Instead of using the provided button to insert AI-generated content, they manually copied and pasted the text. As a result, our tracking system failed to register these articles.
It's also important to say that achieving speed and volume with AI is straightforward—the real challenge lies in refining the quality of AI-generated output. This requires relying on human expertise to iteratively test hypotheses and solve emerging issues. Thankfully, rapid advancements in AI engines have made this easier. In fact, there have been instances where an updated version of the AI solved problems at the language model level before we even had a chance to implement our planned fixes.
4) Measuring as a form of feedback
Every application must be thoroughly measured, especially one designed as a professional tool. We measure how well the system functions and whether it achieves its intended goals. For example, analytics revealed that AI-assisted articles are written almost five times faster than those written manually.
But measurement is more than just speed—it provides valuable insights into user behavior. This allows us to uncover unconventional scenarios and behaviors that could not have been predicted.
For example, we introduced an “AI-assisted” label for articles where at least one paragraph was generated by AI. After a week, we noticed that the number of such articles was lower than expected. It turned out that editors were bypassing the designated path. Instead of using the provided button to insert AI-generated content, they manually copied and pasted the text. As a result, our tracking system failed to register these articles.
5) Local feedback
We are fond of organizing large surveys and conducting interviews, but we have discovered that gathering what we call “local feedback” is also very valuable. It is about gathering feedback in context — right when the user is actively engaging with the system. This approach captures fresh emotions, as the user is still in the moment of their experience.
For example, we know how to identify the exact point when a user finishes their current interaction with a chat. This is the perfect time to ask for quick feedback—a rating and a comment—while the experience is still fresh in their mind.
Some more tips? There you go:
Tip 1: You can ask for feedback at multiple points in the user journey. However, space these questions out to make sure your users are comfortable.
Tip 2: After releasing a new application version, ask the same questions to track how user opinions evolve. This helps measure improvements or identify new areas of concern as the tool develops.
6) Should you give your AI Assistant a name?
There’s a temptation to name AI assistants, just as we name colleagues, pets, or even favorite objects.
Naturally, the idea crossed our minds too, and at first, most of the team was in favor. However, reason prevailed. We’re still in the early phases of using AI, filled with doubts and concerns. We recognize that there are risks associated with AI, but we don’t yet fully understand the extent or impact it may have on the quality of our work. We must remain highly vigilant in evaluating its output. Trusting AI too much would be premature, and giving it a name, thus humanizing it, would only counteract this cautious approach.
Furthermore, regardless of the quality of the AI’s work, it is the human editor who remains fully responsible for the final product. They must thoroughly review, refine, and ultimately sign off on the content. After much discussion, we chose a simple, unambiguous label: AI Assistant. It reflects its true role—assisting, not replacing human judgment. It’s AI, not a person.
Did it spark your interest?
This was the third (and last) part of our insights from the project on implementing an AI assistant. Click here to access Part One (People) and Part Two (Systems). If you feel something needs to be added and want to share a comment, reach out to Slawek, who will happily discuss the subject with you! Also, check out our case studies and recent articles about AI in UX.