A guide to preparing and engaging employees for AI assistant adoption. Part II: System
19 Sep 2024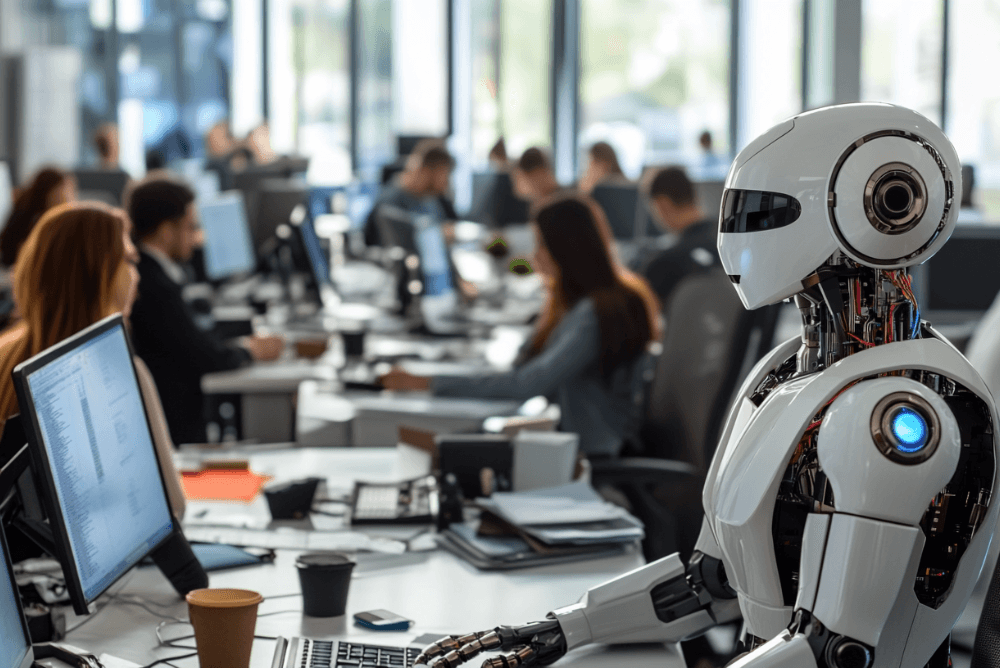
This is the second part of our series of articles, where we share our insights and lessons learned from a recent case study on implementing AI assistants in large companies with 100 to 1,000 employees involved in content creation, as well as in production-focused companies managing thousands of daily service tickets.
We believe our findings can be valuable for both media and production organizations, so if you’re in that loop – jump right in. You will find a short overview of the project and its context here.
This part focuses on the system — highlighting some of the key elements that we believe are crucial for the successful implementation of an AI assistant. The list is not exhaustive – we want to describe what was most crucial (and most challenging to us). These elements are 1) Seamless implementation, 2) Kebab vs. corset approach, 3) AI slowness, and 4) Cost limits.
Also, check out the other two parts of our case: People and Relations.
1) Seamless implementation
Incorporating AI into an existing workflow is not just about technical integration—it’s about creating a seamless user experience. Our goal was to avoid introducing a new app, module, or workspace that users would have to learn from scratch. Instead, we focused on embedding the AI assistant directly into the existing interface, where users already spent significant time each day. This approach helped immediately reduce the distance between the user and the new tool.
In most organizations, the processes AI is meant to support are likely already in place, as are the tools and systems where these processes occur. This was precisely our situation: a well-established editorial process and a customer support system with which our users were familiar. The last thing they wanted was to navigate a new application or adapt to a significant change in their work environment. Therefore, our primary objective was to seamlessly "weave" AI functionality into the existing solutions.
The new features were designed to leverage familiar patterns and components from the existing design system, making the anticipated revolution in their workflow feel more like an evolution of their current application.
This approach not only made the transition smoother for users but also accelerated the implementation and reduced costs associated with training, support, and system maintenance. However, it's important to note that while seamless integration is key for final deployment, we recommend conducting experiments and Proofs of Concept (POCs) separately. Rapid prototyping, quick testing, and fast iteration can lead to valuable insights, which then inform more strategic, long-term decisions for full-scale implementation.
Watch: 6 non-obvious signals showing you might need UX consultancy:
2) Engine agnostic approach
Steve Jobs once said, "First, customer experience, then technology."
Following this philosophy, we adopted an engine-agnostic approach from the very beginning. Our goal was to ensure that the underlying technology or engine powering the AI assistant remains invisible to the user.
Whether the engine changes or evolves over time, the user experience stays consistent, with no need for adjustments on their part. This transparency ensures that the focus remains on usability and functionality, without burdening users with technical complexities.
3) Kebab vs. corset approach
When it comes to implementing AI in a company, multiple departments are involved in the decision-making process. At the table, you'll find representatives from IT, finance, and legal teams, each bringing their own set of constraints. We refer to those constraints as the “corset” approach—a model that shapes the final tool by tightly restricting its form.
The corset is restrictive, rigid, and allows for only a predefined shape, which can stifle innovation, creativity, and bold visions of the future. While this approach might keep the project within safe and predictable boundaries, it often hampers the very potential that AI can unlock.
We prefer a different method—one we call the "kebab" approach. In this scenario, we start by sketching out an ideal, bold, and unrestricted plan. At the core of this vision are business objectives and user needs—nothing more, nothing less. This approach allows for the fullest expression of creativity and innovation, focusing on what could be achieved in an ideal world without immediate constraints.
Only after crafting this ideal vision do we start layering on the necessary constraints from IT, finance, and legal. We carefully trim away elements that are too difficult, too expensive, or too legally risky. Given how rapidly technology and legal-financial conditions are evolving in the realm of AI, I guarantee that the “kebab” approach will yield far more valuable results than the restrictive corset.
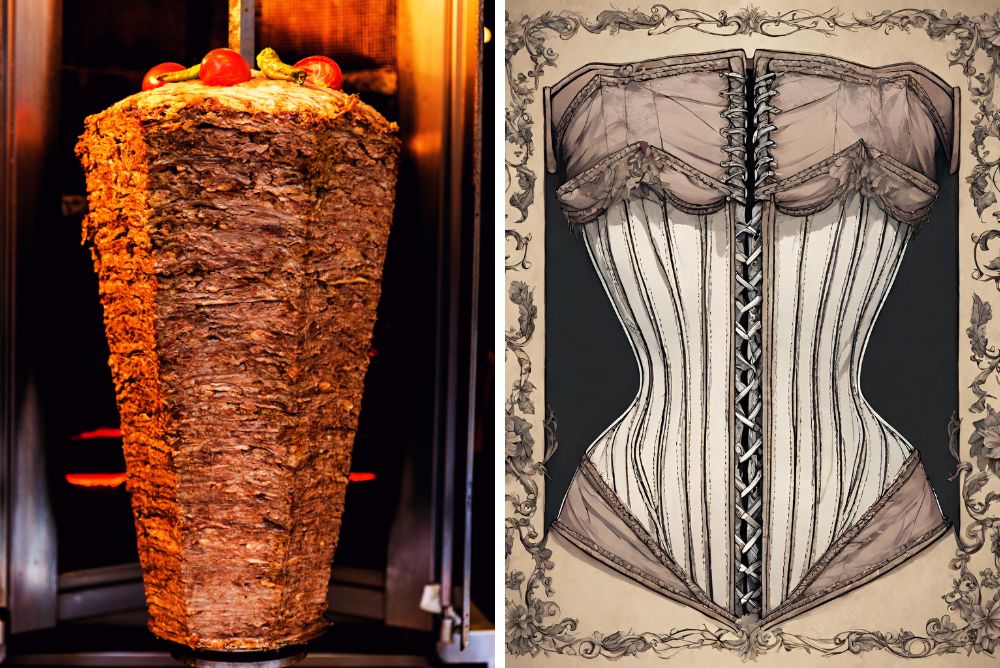
Kebab and corset are names for approaches towards decision-making process.
4) AI can be slow
At first glance, AI impresses with its speed. Tasks that used to take minutes, hours, or even days are now completed in seconds. This rapid response time is one of the key attractions of AI, creating a sense of efficiency that quickly wins over users. However, the daily, widespread use of AI in a large company can also bring less favorable experiences.
While AI is generally fast, there will be moments when response times are significantly longer than expected, testing even the most patient users. This can be frustrating, especially when users have grown accustomed to the usual speed of AI. It's important to anticipate these delays and manage user expectations effectively.
There will also be instances where AI fails entirely, and the expected results simply do not materialize. This could be due to system errors, overloaded servers, or limitations in the AI's capabilities. These moments, though rare, can erode trust in the system if not handled properly.
Read also: Work, future, and AI: The three realms ahead of us
How to prepare your system
To mitigate these challenges, it's essential to build a robust framework that can handle both delays and failures effectively.
Informative process updates
A simple spinning wheel with a "loading" message is not enough. After about 10 seconds, users will start to think the system has frozen. To prevent this, implement a multi-stage progress update that changes over time, reassuring users that the AI is working and moving through different phases of the process. This not only keeps users informed but also increases their tolerance for waiting.
Process interruption options
Sometimes, users may not want to wait or might be dissatisfied with the initial output. Providing an option to stop the process can save time and allow users to make an informed decision about the next step, such as restarting the AI or choosing a different approach.
Manual mode
Whenever possible, prepare a backup plan—a manual mode where the task can be completed entirely by a human. Just like people, AI can have its off days, and having a manual option ensures that work can continue without major disruptions. This fallback option is crucial for maintaining productivity and user confidence.
5) Cost limits
We need to say it: AI comes with its own set of costs. As the scale of operations grows, the cost of using AI engines becomes significant, and without careful management, you could find yourself in a situation where the expense of AI-assisted work approaches the cost of having a human do the job.
For example, we realized that working with longer texts or refining their quality through multiple iterations means that the number of tokens used increases almost exponentially. This is because the AI often needs to resend chat history or source content to maintain context. This can quickly drive up costs if not kept in check.
Implementing safeguards and educating
To avoid spiraling expenses, you need a robust system for cost control. Start by creating a cost forecast for AI usage within your processes. Define a break-even point for the entire operation as well as for individual tasks. This will help you understand when AI remains cost-effective and when it may start to become too expensive.
In our experience, setting a limit on the number of prompts allowed per content piece proved effective. For example, capping it at 10 prompts per article helped us maintain cost-efficiency without compromising on quality. This kind of fuse is essential, especially when dealing with a large user base, where costs can quickly escalate.
6) Knowledge base
When introducing a new tool like an AI assistant, it’s common for users to feel resistance, often due to the learning curve and the fear of disruption to established workflows.
To address potential user concerns, and avoid any confusion about where to seek support after the initial implementation phase, we implemented a four-layer knowledge system for our AI assistant:
Centralized instruction manual: A comprehensive guide outlining the rules and guidelines for using the AI assistant, as well as broader AI practices within the organization.
Contextual instructions: In-app prompts and explanations tailored to specific features and options, providing real-time assistance.
Update policy: Recognizing the challenge of keeping documentation current, especially for rapidly evolving tools, we have assigned dedicated personnel and processes to ensure the documentation stays up-to-date.
Human support: The final layer of support, which includes designated contacts such as AI ambassadors who have direct communication with IT. This structure ensures that issues related to the language model, often beyond IT’s expertise, are addressed by refining prompts based on editorial needs.
Read also: How to build a knowledge system around a new tool?
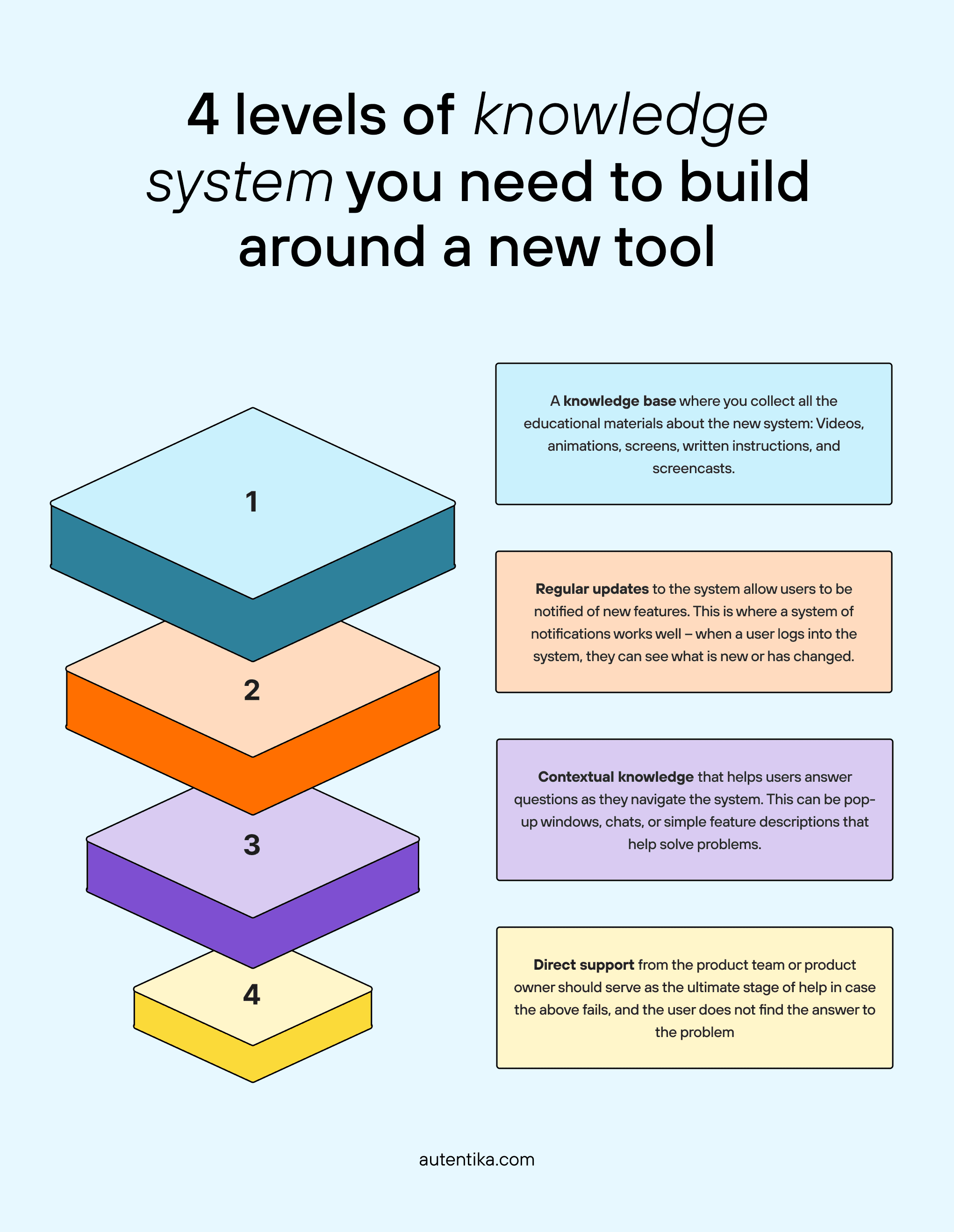
7) Preparing for the unknown
Given the current pace of AI development, we face an increased risk of technological debt emerging just weeks or months after implementation. This amplifies the already high importance of readiness for change, flexibility in our processes, and adaptability in the interface managing it all.
When we design a process, we constantly ask ourselves, "This works for now, but what if tomorrow...?" Ideally, our implementation plan follows an evolutionary path—each new version builds upon and improves the existing tool rather than introducing entirely new systems. This approach ensures users remain familiar with the tool as it evolves, minimizing disruption. Instead of discarding the MVP (Minimum Viable Product), we aim to use it as a foundation to continually add new layers and capabilities.
Read also: What’s the gold standard for a discovery process? See our exemplary approach – BBUD
Keep reading!
This was the second part of our insights from the project on implementing an AI assistant. Click here to access Part One (People) and Part Three (Relations). If you feel there’s something missing and want to share a comment, reach out to Slawek who will happily discuss the subject with you!