Automating the impossible: Jesse Lord on Fabriq
31 Jul 2024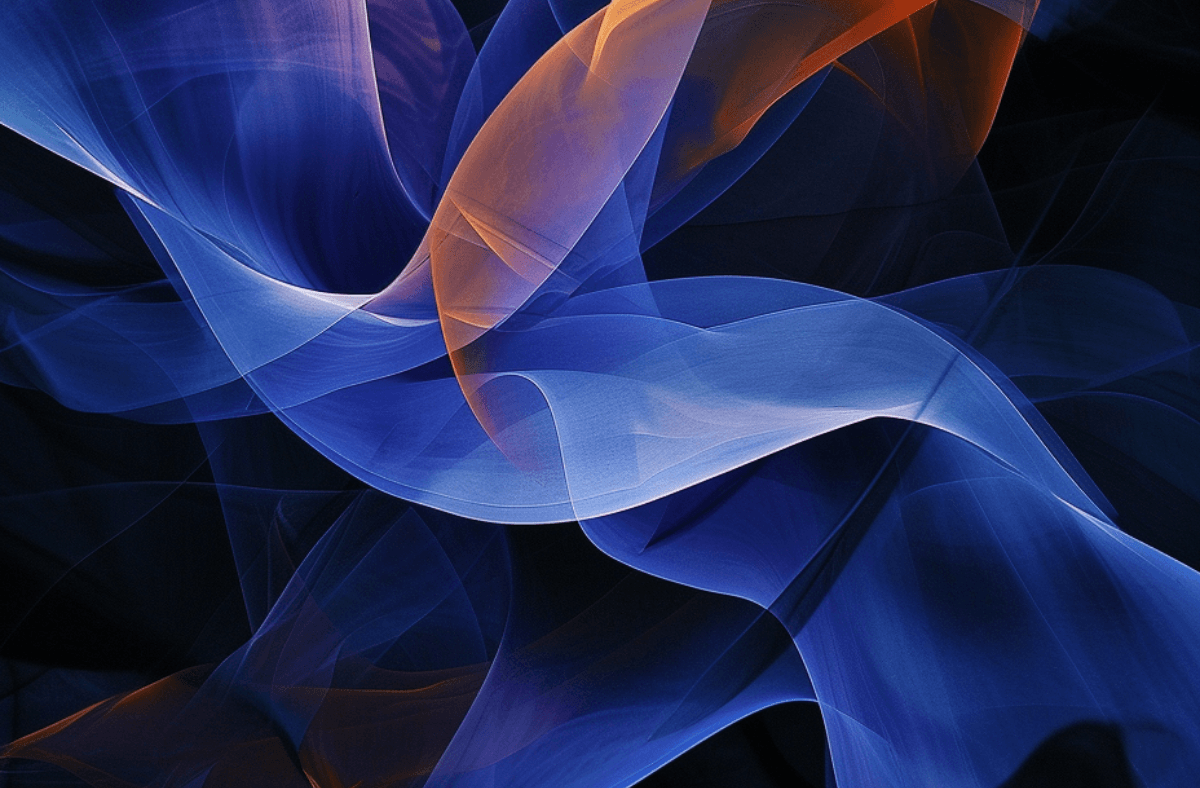
We interviewed Jesse Lord, Product Strategy Lead at KADME and co-founder of Fabriq, a new automation platform that leverages LLMs to optimize processes.
Unlike general search engines that often return irrelevant results, Fabriq delivers accurate, contextualized information. Its conversational interface streamlines workflows, helping companies leverage their extensive investments in detailed reports by building on decades of accumulated knowledge.
Autentika: Hello Jesse! Can you explain the primary objective of the Fabriq platform
Jesse Lord: Our main goal is to automate or semi-automate parts of businesses using advanced technology. The platform has evolved significantly with the development of new AI models and we now use these technologies to automate processes in ways that were impossible only a year ago.
How do you approach the initial stages of a project with a new client, and how does Fabriq ensure that the chosen AI solutions meet their specific business needs?
When we start a project, we receive client documentation and data samples. This documentation includes problem statements, and our task is to match the business challenge with the data and current technology. We conduct a feasibility study to see if our tech solutions can meet the client's needs and whether Large Language Models can solve practical problems.
Fabriq’s platform can process client data to address specific industry-related queries. For instance, in the case of an industrial machine manufacturer, the query might be about adjusting a specific function in a machine. Fabriq searches for relevant information amongst their documents, diagrams and databases, then contextualizes it and generates useful answers. It’s not just answer generation though; it might be more valuable to focus on automation opportunities such as creating qualified service requests based on diagnostic data. This process helps us meet the customer's objectives and justify moving forward with a feasibility study.
In this context, does AI enhance the effectiveness of sales and customer service teams, or does it become an independent subject matter expert?
For many companies, AI can serve as a supportive helpdesk. Traditionally, a sales representative might not memorize every detail of every instruction, so they search technical manuals to provide answers. AI can automate these tasks, offering quick, accurate product information. Sales reps or customer service specialists can then focus on tasks that demand human interaction.
On the other hand, engineers retain detailed technical information but might not engage well with customers. AI bridges this gap, providing salespeople with technical information in natural language. Our specialized AI delivers precise answers tailored to user needs, enhancing sales team effectiveness without replacing the human element.
What distinguishes Fabriq’s specialized AI from general chat AIs, and how does your approach ensure response accuracy and relevance?
The key difference is context. General chat AIs, like the initial version of ChatGPT, often lack specific knowledge about a product and provide vague or incorrect information. In contrast, our platform collaborates closely with the customer, using data they provide and consulting with subject matter experts.
Additionally, with models like GPT-4o, which are natively multimodal, we can handle audio, images, video, and speech simultaneously, enhancing the versatility and effectiveness of our AI.
Read also: The future of AI in sales: Insights from SMOC's CEO, Kristoffer Kvam
Can you give an example of a specialized AI solution you’ve developed and how it caters to the specific needs of its users?
For instance, we provide the platform that powers AtlasGPT, a solution dedicated to neurosurgeons, initiated by Dr. Cohen, who is incredibly knowledgeable and passionate about his field. He has helped us understand what's important to him and his customers.
AtlasGPT uses cutting-edge retrieval-augmented generation (RAG) techniques to ensure the highest standards of output. This approach combines the generative strength of large language models (LLMs) with an external data retrieval mechanism. The system retrieves relevant documents from sources like the PubMed, the Journal of Neurosurgery, the Neurosurgical Atlas, and the Principles of Neurosurgery, extracts key information, and uses that to guide response generation. This enables personalized, factually consistent responses without exposing private data.
AtlasGPT employs digital personas (assistants) to provide detailed answers about neurosurgical procedures. The idea is to have a digital personality you interact with, offering answers with varying levels of depth and complexity. For example, you can ask about the outcomes for hemicraniectomies in stroke patients after mechanical thrombectomies and receive responses ranging from highly technical medical jargon to explanations understandable by patients.
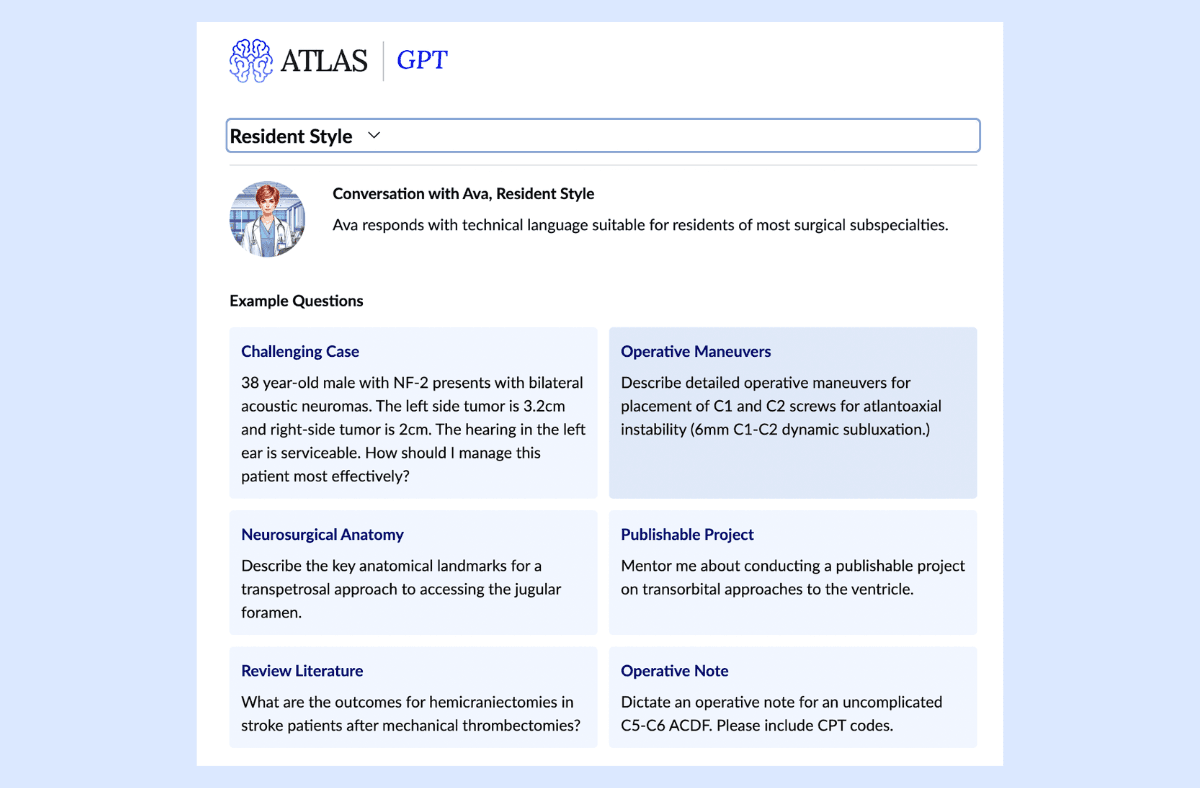
AtlasGPT is a solution dedicated to neurosurgeons that uses retrieval-augmented generation (RAG) techniques to ensure the highest standards of output.
How do you ensure the trustworthiness of data sources used to feed the platform?
In AtlasGPT, we only use peer-reviewed sources like the Journal of Neurosurgery and other trusted sources such as PubMed. While peer review is a hallmark of reliability, not every article is infallible. Verifying the accuracy of information, especially when using language models like GPT4, is crucial. Our approach leverages language models for summarization, with qualified individuals vetting the information to ensure accuracy and transparency.
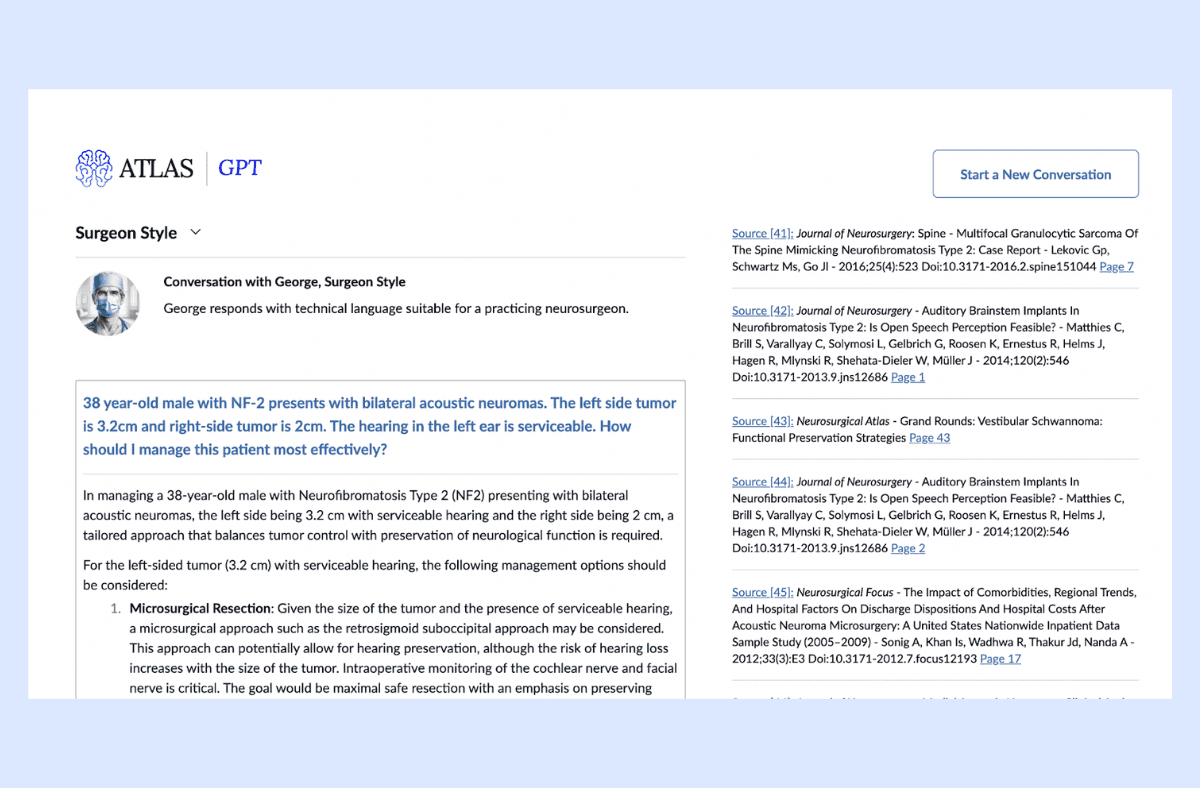
AtlasGPT uses peer-reviewed sources like the Journal of Neurosurgery and other trusted sources such as PubMed.
How do you select and manage datasets to feed the model? Is it done automatically?
We can integrate with a variety of data sources and types. Documents, presentations, databases, spatial data, journals and XMLs typically serve as data sources in our projects. Having a data platform in the mix such as Kadme’s Lumin can also really help with integrating with private data at scale. We can extract images from PDFs, classify them, and integrate this data through our API, ensuring seamless integration with various interfaces. For larger datasets, like 268,000 images in one project, we build and deploy a model that classifies images and documents. This model can be updated through user feedback and retrained based on incorrect outputs. Built-in metrics and visual evaluations help identify and correct issues. The main point is to avoid solutions that require the continuous employment of expensive data scientists.
Who becomes the owner of the data fed to the AI?
While this bucks AI industry trends, we’ve found enterprise clients expect data privacy and ownership. With Fabriq, customers own both the data that goes in and the generated output. Since the output is created using their private data, it is considered a derivative work that is also their property.
How do you approach educational bias in models? Does this bias exist, and what can be done about it?
Bias is inherent in all models due to human judgment calls in labeling. Different cultural influences can lead to different biases. For example, reinforcement learning conducted in San Francisco will produce different results from reinforcement learning done in Beijing due to cultural differences and biases in labeling.
A classic example from machine learning involves an AI model used to determine if a prisoner was likely to reoffend on parole. Initially, the model achieved high accuracy by using the prisoners' ethnicity as a primary feature, revealing racial bias. Removing ethnicity as a feature led the model to use zip codes instead, which was just a different expression of the same bias. In this case the model is unable to see the underlying systemic causes of these outcomes, as they are not represented in its training data.
For instance, European legislation on AI considers it a high risk to have systems that can infer human emotions or predict who might commit future crimes, aiming to limit the adverse effects of biases in AI applications.
What does the implementation of Fabriq mean for the end user? What results can a company expect, let's say, in 2 years' time?
The outcomes vary depending on the customer. For example, the Norwegian Offshore Directorate has enabled natural language interactions with their relinquishment reports, supporting the national offshore license round process. Atlas Meditech has created a new product, soon commercially available to its members as well as other businesses and institutions within its industry. Glex and Kadme use Fabriq as a plugin to provide natural language interactions with private data as an integrated part of their software offering.
Read also: Fact-checking in the age of AI: no longer just for newsrooms
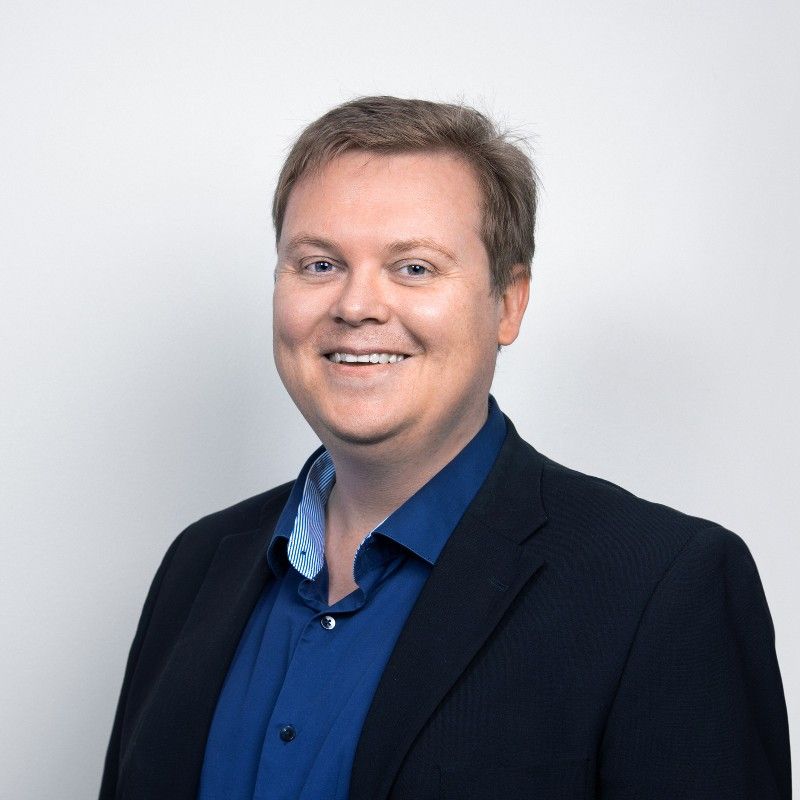
Jesse Lord, Product Strategy Lead at KADME and co-founder of Fabriq.