AI in retail – what’s still possible?
29 Feb 2024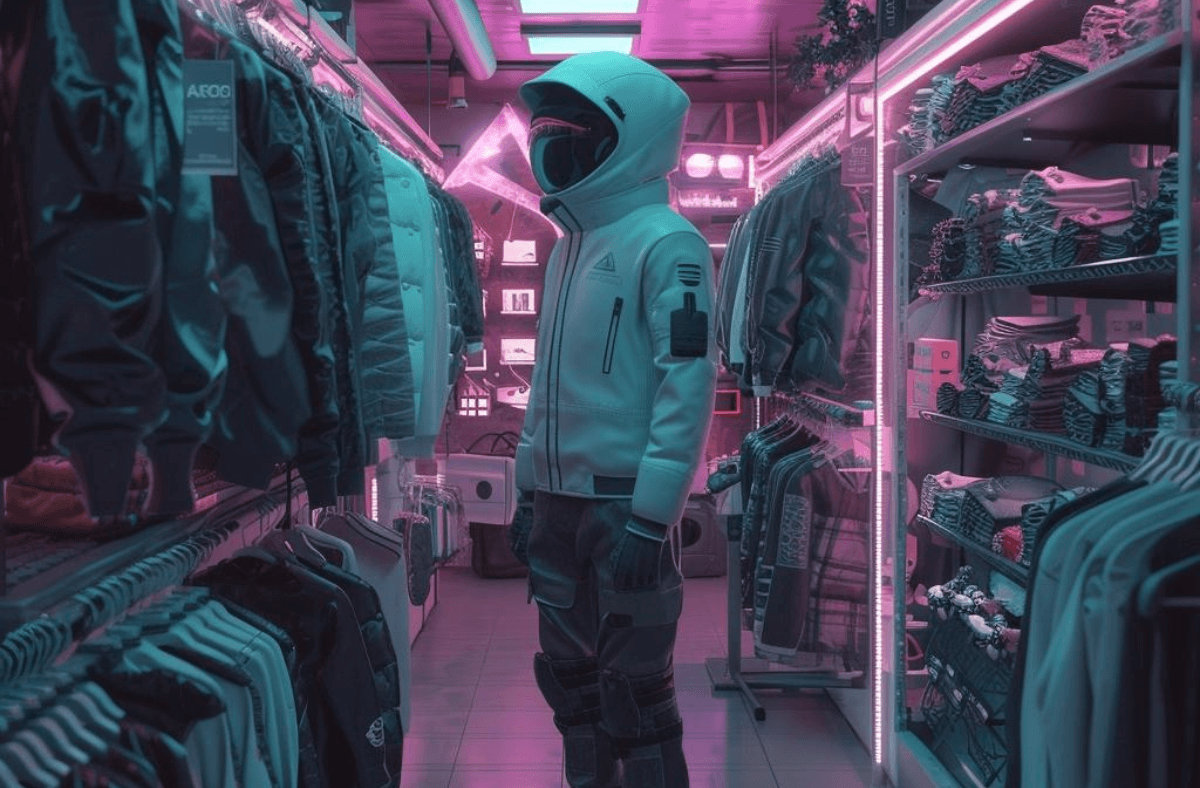
Online retailers have enjoyed a remarkable advantage and a head start with AI. Their established processes have allowed them to collect data by default, and they have been using analytics to optimize the customer experience for years. But what about the future? Where will AI take retail, especially in enhancing back-office operations and processes? And does it have the potential to enhance just online stores or traditional brick-and-mortar shops, too?
Over the past five years, the landscape of AI in retail business applications has undergone a remarkable transformation. Back in 2019, personalization was limited to basic recommendation engines relying on past purchases, and chatbots offered simple customer service functions. Today, advanced AI algorithms enable hyper-personalisation, analyzing extensive datasets to craft tailored shopping experiences, targeted promotions, and dynamic pricing. Conversational AI bots handle complex inquiries and negotiate prices, and even physical stores utilize AI-powered cameras and sensors to track customer behavior and personalize in-store experiences.
But what about internal business apps, operational and back-office processes? How can AI help retailers streamline their workflows and cut costs? The potential seems tangible: AI can increase operating margins by up to 4 percentage points and commercial margins by up to 6 percentage points, as shown by the analysis by Oliver Wyman. And the research by Deloitte proves that 91% of all organizations expect their productivity to increase due to generative AI. Many of them are in the process of redesigning their business operations, ensuring both adoption and ethical use, but the overall growth in the adoption is slow.
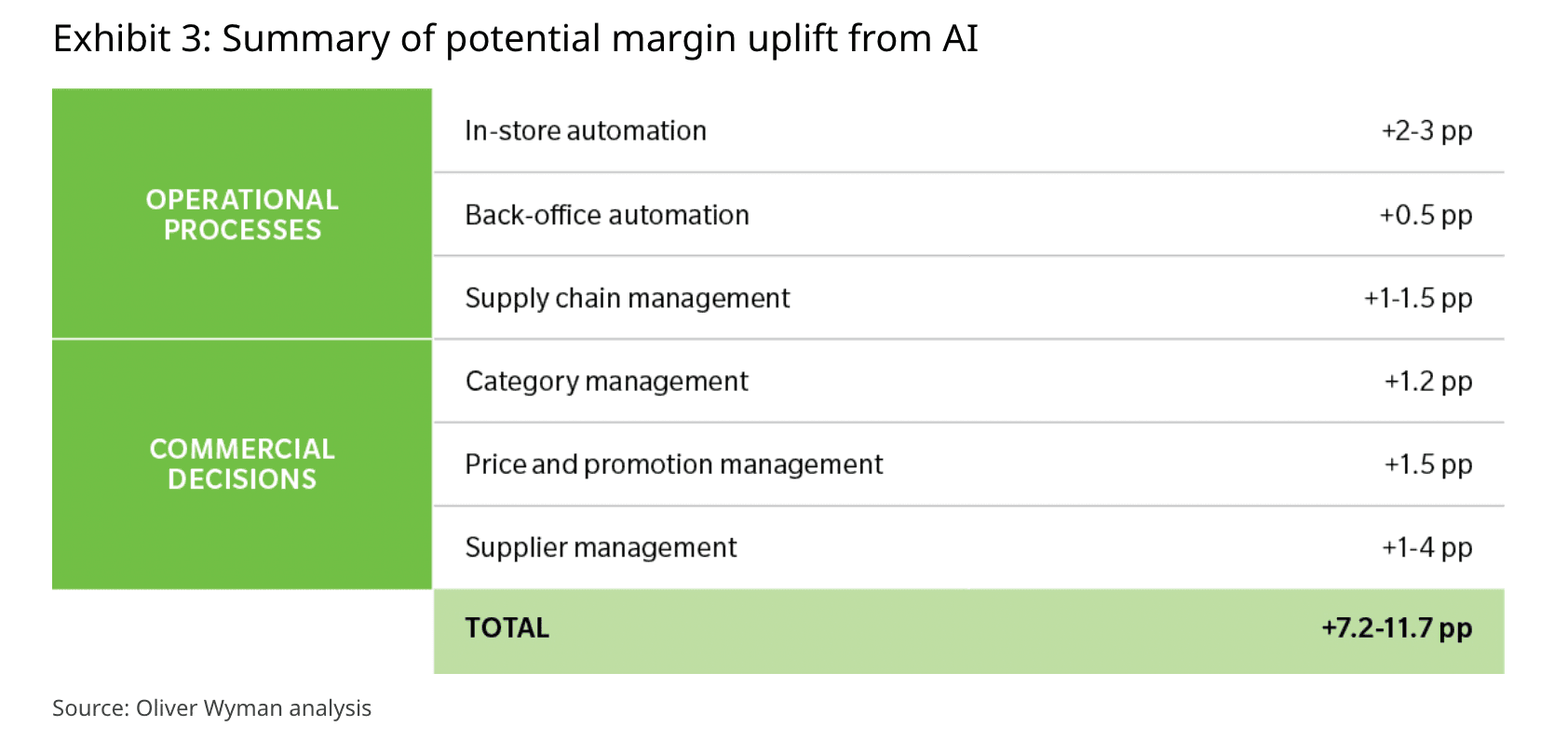
Let’s take a closer look at these categories and examine how industry leaders use this technology in their retail strategies.
1) In-store innovation and automation
The impact of AI on e-commerce and online stores is obvious. But what about traditional retail stores? Here, AI can help with inventory placement, pricing and matching products to in-store customers. More and more stores – especially those selling clothing, shoes and accessories – can track how often an item is picked up, tried on or left in the fitting room. This data collection helps identify customer behavior and – like in an online store – capture impressions, intentions, abandoned purchases and sales.
AI can also significantly reduce the workload of sales staff and take over some repetitive tasks such as replenishment and checkout. Automated checkout goes beyond the self-checkout systems commonly found in supermarkets, as it recognizes items placed in a basket without the customer or an employee having to do anything. The simplest systems register when a customer takes items from the shelves and then deduct the total amount from a digital payment system.
By the way, the same happens in online stores. Amazon Go is one of the first examples, using ‘just walk out shopping’ technology that reacts to products taken from the shelf and invoicing them to the customers' Amazon account. This technology has also been used by other retailers, such as Alibaba and Aldi, and Żabka.
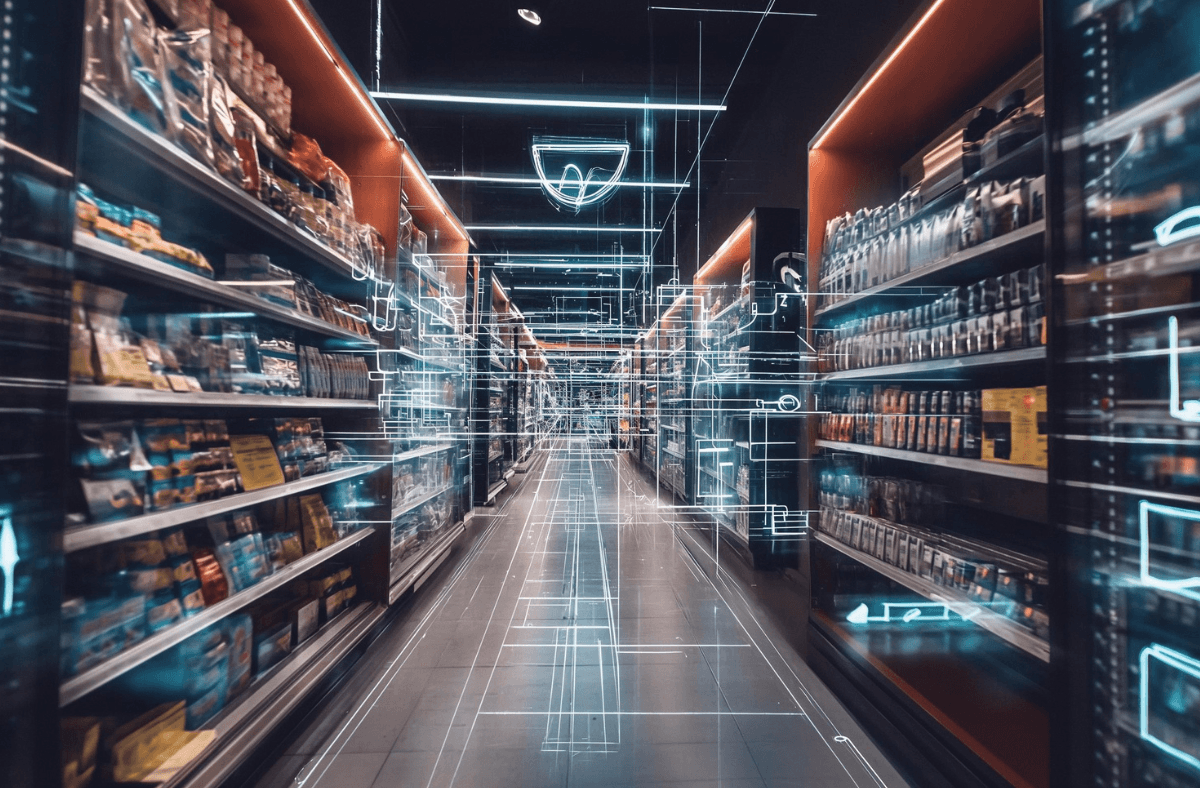
"Just walk out shopping" is a technology that reacts to products taken from the shelf and invoiced them to the customer's digital wallet (image by Midjourney).
2) Back-office automation
AI-driven tools offer a robust solution for automating various back-office tasks. For instance, the technology can efficiently process invoices and execute payments, even when dealing with non-standard paper formats. Early errors are part of continuous learning, contributing to the system's self-improvement over time.
Experts forecast that this level of automation will reduce the headcount of entry-level accountants and other back-office staff and enable senior management to react faster to changing business conditions.
Considering that back-office operations typically represent around 1 p.p. of a retailer's costs at headquarters, the implementation of AI has the potential to increase the company's margin by 0.5 p.p. (forecast by Oliver Wyman).
3) Inventory and supply chain management
Most retailers have already digitized – at least to some extent – their distribution centers. Orders are placed automatically, and robots move pallets around warehouses. AI instructs a warehouse where to stack boxes in a truck so that they are automatically unloaded in the right order. Human shelf stackers then don’t have to search or think so much.
Still, AI can further reduce supply chain costs and boost efficiency, as advanced analytics can figure out the cheapest, quickest routes and consider a wider range of factors than a person can. While supply chain costs are generally equivalent to just 2 to 3 percent of sales, AI in this area could potentially boost a retailer’s margin by around 1.0 to 1.5 p.p., according to Olivier Wyman.
AI and machine learning mechanisms also prove efficient in supply chain forecasting, allowing for accurate demand forecasting and improving planning processes across merchandising, supply chains, and operations.
Here's an example of how an app designed for parcel courriers improved route planning . Since a courier delivers dozens or even hundreds of parcels every day, route optimization is crucial, as it helps save time and gas costs.
Another example is a UK convenience business, One Stop, that adopted such solutions in 2022, reporting significant improvements after four months of using an AI forecasting solution. This included a 1.9% increase in in-store availability and a 4% reduction in fresh spoilage value, contributing to a significant sales increase.
4) Price optimization
Many retailers still plan their promotions around intuition – but when we put aside “what everyone does” and employee AI to reveal the true impact of promotions, we can arrive at astounding conclusions. Thanks to advanced mechanisms, it’s possible to measure the impact of promotion against other data – and see that, in some cases, promotion might harm the business, not leading to other sales that compensate for the costs.
According to Oliver Wyman, a sophisticated promotion algorithm can increase the company’s margin by 1.5 p.p. over one or two years.
Amazon has been the pioneer of these strategies for many years. The retailer adjusts product prices in real-time based on demand, competition, and market factors, maximizing revenue and setting trends.
5) Content generation
Retail is a lot about content generation – both in marketing campaigns and product descriptions. This is where the power of Generative AI can be at its fullest, and there is no need to use external tools such as ChatGPT – retailers can have their own AI-assisted content management systems and use AI to generate all types of content – from email marketing campaigns to product information.
The AI features can look similar to the editorial tools used by journalists in newsrooms, as shown in this proof of concept, designed by Autentika. AI generates a paragraph based on a given topic, with the tone of voice and word count specified by the user.
The next step here is creating more personalized content. For example, Starbucks generates personalized email newsletters with customized offers and recommendations based on customer preferences and purchasing history, while Nike publishes personalized product descriptions.
Another benefit of using AI in content generation is consistency – thanks to algorithms, you ensure that product descriptions are accurate and standardized, making products more discoverable.
With Gen AI, it’s also possible to generate personalized product visuals. Here, IKEA is a good example – with the company’s AI-powered system, customers can get customized rooms and apartment visualizations with product recommendations. The visualizations are made based on customer preferences and room dimensions, ensuring high accuracy.
Building AI-assisted tools for retail
According to the recent Deloitte’s State of Generative AI in the Enterprise report, most organizations rely primarily on off-the-shelf generative AI solutions for now. These include productivity applications with integrated generative AI, enterprise platforms with integrated generative AI, standard generative AI applications, and publicly available large language models (LLMs), such as ChatGPT.
Relatively few use more customized AI solutions, such as industry-specific software applications. However, it is expected that as AI becomes more specialized and strategic, we will see more complex use cases of business apps tailored to the needs of specific industries and companies. Many organizations will have to decide whether to build solutions in-house or hire external consultants. If you're interested in which solution migh be better for you, check out this article.