Why do AI projects fail?
4 Nov 2024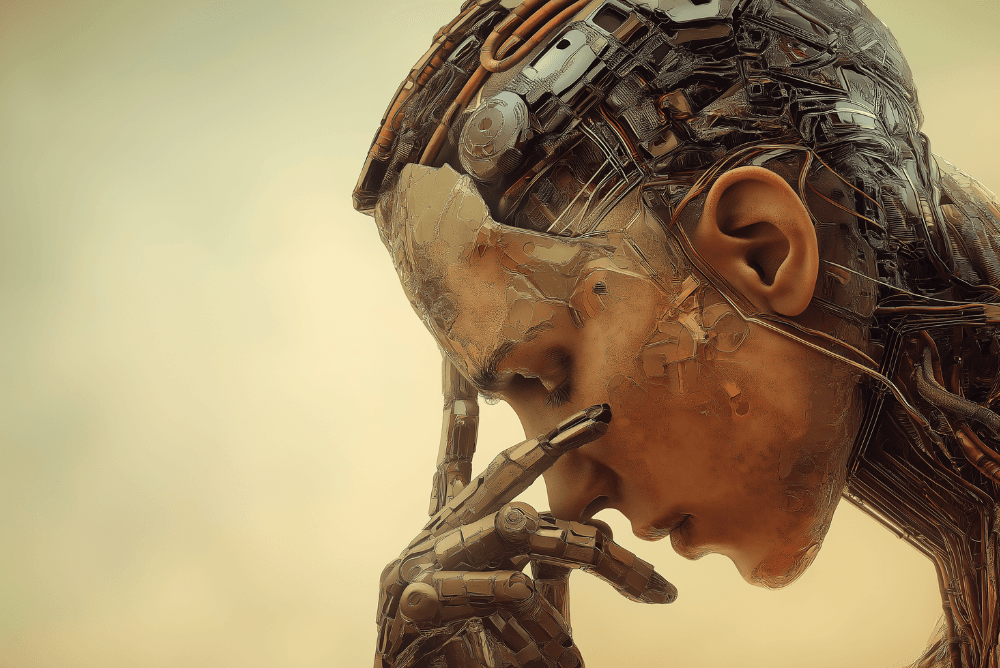
AI projects have an exceptionally high failure rate – and that’s not clickbait. According to numerous studies and surveys, failure rates for AI projects can reach as high as 80%, almost twice the failure rate of traditional IT projects. Let’s investigate some of the reasons why it happens.
A recent Deloitte study showed that only 18% of organizations reduce costs thanks to AI, and only 27% improve efficiency and productivity. Back in 2021, Gartner alarmed that just 53% of AI projects transition from prototype to production – mainly because CIOs and IT leaders find it hard to scale AI projects due to the lack of tools to create and manage a production-grade AI pipeline. Research has shown that this “pilot paralysis,” where companies launch pilot AI projects but fail to scale them, is surprisingly common.
At the same time, experts are convinced that most failures could be avoided – if only business leaders grounded their projects in achievable outcomes. Harvard Business Review once called this disconnection between expectations and reality “dumb reasons” – but are they really that dumb?
Let’s look at some statistics and primary causes of AI project failure based on recent studies and data.
1) Unrealistic expectations
One of the most common reasons for AI project failure is unrealistic expectations about the technology’s capabilities. Many managers mistakenly expect AI to solve complex problems immediately without understanding its iterative development nature. When management "thinks too big", the scope of the project often expands beyond what is realistically achievable.
What’s also crucial is that AI projects are sometimes only entitled as AI but are, in fact, not using any AI-related technologies. These projects can be considered failures since they did not really lead to AI adoption.
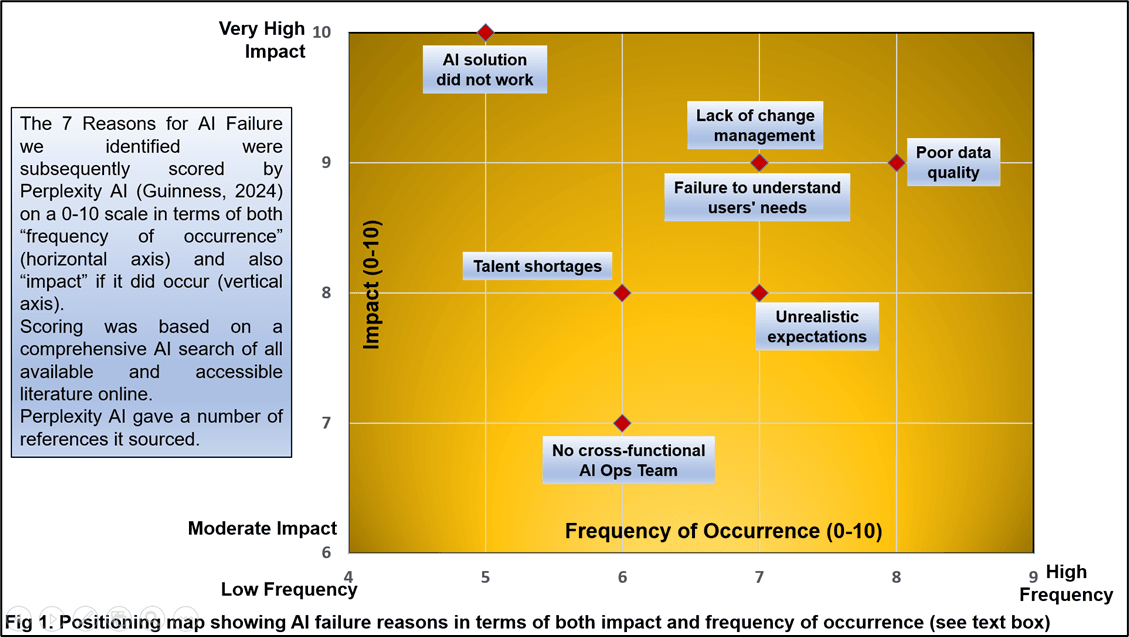
Screen from Why AI Projects Fail: Lessons From New Product Development by Robert Cooper.
2) Failure to understand users’ needs
Many organizations fall into the trap of adopting a technology-first approach, which means they focus on the allure of AI technology without first investigating specific problems that need solving. As we have repeatedly said in our publications, it’s best to begin with a clear understanding of the user problem and only then decide which AI solution fits.
Read also: What’s the gold standard for a discovery process? See our exemplary approach – BBUD
A McKinsey report from 2023 emphasizes that misalignment with user needs is a major reason for AI failures, much like in new product development (NPD). When organizations fail to conduct adequate user research and don’t involve end-users during development, the solutions created often miss the mark. Without an understanding of users' pain points, workflows, and applications, even the most advanced AI solutions risk being irrelevant or poorly integrated into existing processes. This leads to poorly defined project objectives and ultimately contributes to high failure rates.
3) Poor data quality
AI systems rely on high-quality, relevant, and properly labeled data for both training and operation, so if the quality of the input is poor, it will lead to unreliable models. In Deloitte’s survey, 33% of respondents cited a lack of confidence in results as one of Generative AI’s top risks (third in the list of top 10 risks).
Many companies struggle with a solid data infrastructure, making it difficult to gather or manage the necessary information. As written in the Deloitte report, “Trust related to data quality, LLM training, and output reliability becomes particularly important”.
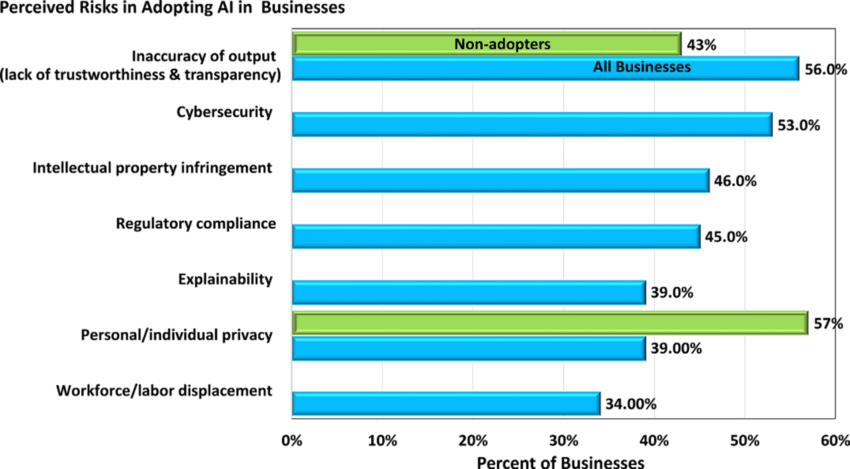
Percentage of businesses citing each risk in the adoption of AI in their business (sources of data: McKinsey 2023; IBM Newsroom 2024)
4) Talent shortages and lack of resources
Implementing AI is resource-intensive, requiring not only data but also skilled people. In one study, respondents said that the lack of expertise was a key reason for the failure of AI projects. As one of the interviewees put it in one of the studies, “If you put the wrong person, a person without enough knowledge, on an AI project, it is possible that the budget gets blown without any outcomes.”
Organizations often underestimate the skilled human capital required to execute AI projects successfully, leading to understaffed initiatives. This lack of resources results in either stalled or abandoned projects, a phenomenon sometimes referred to as “pilot paralysis,” where companies get stuck in perpetual proof-of-concept phases.
5) Organizational constraints and misalignment
Budget limitations, organizational silos, and internal resistance to change can also derail AI projects. Many organizations set budgets that are too low or fail to align AI initiatives with broader business strategies, creating friction between departments. Additionally, regulations or compliance issues can hamper AI development, especially in highly regulated sectors like healthcare or finance.
Robert Cooper’s report noted that AI projects require collaboration across various functional areas. When teams don’t work together, it results in misalignment and failure to integrate AI solutions into existing business processes. Others agree with him, too. “Having an AI Ops team composed solely of technical personnel, such as data scientists and IT-techs, is likely to result in solutions that are disconnected from real user needs and operational realities”, writes Cem Dilmegani, Principal Analyst at AIMultiple in his article ‘4 Root Causes to Avoid for AI Project Failure”.
6) Technological obstacles
While AI is often seen as a cutting-edge solution for an organization, the underlying technology can pose significant challenges, especially if a company has a legacy of old tech infrastructure and a tech debt hindering digital transformation efforts.
One study shows that the top reason for AI failure is that the model “did not perform as promised”. The causes behind it include some of the aforementioned factors such as inadequate data quality, algorithmic limitations, and model instability (as algorithms are updated, the new system may not give the same results as the previous one). Another reason is that AI algorithms lack transparency. This is the issue known as the “black box” problem (it is difficult to understand how an AI system arrives at its conclusions or predictions).
Read also: 6 non-obvious signals showing you might need UX consultancy
7) Lack of change management
Finally, we have to mention the importance of change management when implementing AI solutions. AI is not just a technical challenge, but a cultural one. Companies that fail to prepare their teams for the changes AI will bring often face resistance, which can derail even the most promising projects. Talent shortages, combined with poor change management practices, exacerbate this issue, making it difficult for teams to fully integrate AI into their operations.
Change management is also important because employees have and will have fears and phobias concerning AI. Some of th primary fears are (division, suggested by Junesoo Lee in AI as “Another I”: Journey map of working with artificial intelligence from AI-phobia to AI-preparednes”)
- AI-literacy: the sentiment of “I don't know how AI works or how to use it”;
- AI-substitutability: “I am afraid that AI will make me obsolete and replace me.”
- AI-implementability: “I have to adopt AI just because I have to do so.”
- AI-accountability: “When something goes right or wrong, who or what is responsible: me or AI?”
People’s anxiety towards AI stems mainly from information asymmetry concerning AI mechanisms and impacts. AI designers and developers in the company may have a different knowledge level than end-users, who lack an understanding of AI’s workings (because of the black box issue). Also, with AI's accuracy, speed, breadth, and depth surpassing humans in many intellectual activities, people question their competitiveness in the labor market. Employees are also unsure about unclear accountability for decisions and performance supported or conducted by AI (If AI-provided information is incorrect, who is responsible: the programmer, user, or AI?).
Read also: How to build a knowledge system around a new tool?
We have discussed the process of preparing and engaging employees in AI adoption in detail in a special series of articles: “A guide to preparing and engaging employees for AI assistant adoption”. Check out all three parts we divided it into: Part I: People, Part II: System, and Part III: Relations.
Do you need help in understanding users’ needs?
AI holds enormous potential, but its implementation comes with many challenges. We can help you with user needs and expectations, UX research, and preparing the organization for digital change. We have a proven process and track record to guide you through every step of your digital transformation.
Ready to explore how we can help you overcome your AI challenges?
Contact us to discuss your specific needs, and don’t miss our latest case study on implementing an AI-assisted chatbot in a manufacturing company's customer service department.